Application of Graphs in a One Health Framework
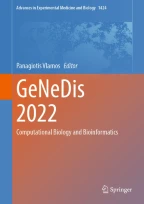
The One Health framework, which advocates the crucial interconnection between environmental, animal, and human health and well-being, is becoming of increasing importance and acceptance in health sciences over the last years. The hottest public health topics of the latest years, like zoonotic diseases (e.g., the recent pandemic) or the increasing antibiotic resistance, characterized by many as “pandemic of the future,” make the more holistic and combinatorial approach of One Health a necessity to combat such complex problems. Multiple graphs and graph theory have found applications in health sciences for many years, and they can now extend to usage across all levels of a One Health approach to health, ranging from genome, one disease level, to epidemiology and ecosystem graphs. For that last ecosystem layer, a proposed approach is the utilization of process graphs from the chemical engineering field, in order to understand a whole system and what constitute the most crucial aspects of a One Health issue in ecosystem level. Here P-graphs are focused alongside their combinatorial algorithms, implemented in R, and their application researched in an effort to extract information and plan interventions.
This is a preview of subscription content, log in via an institution to check access.
Access this chapter
Subscribe and save
Springer+ Basic
€32.70 /Month
- Get 10 units per month
- Download Article/Chapter or eBook
- 1 Unit = 1 Article or 1 Chapter
- Cancel anytime
Buy Now
Price includes VAT (France)
eBook EUR 213.99 Price includes VAT (France)
Hardcover Book EUR 263.74 Price includes VAT (France)
Tax calculation will be finalised at checkout
Purchases are for personal use only
Similar content being viewed by others
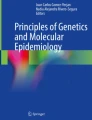
Systems Medicine Applied to Epidemiology
Chapter © 2022
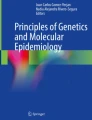
Principles of Network Models and Systems Epidemiology
Chapter © 2022
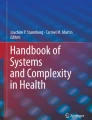
Understanding Complex Systems
Chapter © 2013
References
- Aytan-Aktug, D., Nguyen, M., Clausen, P., Stevens, R. L., Aarestrup, F. M., Lund, O., & Davis, J. J. (2021). Predicting Antimicrobial Resistance Using Partial Genome Alignments. mSystems, 6(3), e0018521. https://doi.org/10.1128/mSystems.00185-21
- Bath, P. A., Craigs, C., Maheswaran, R., Raymond, J., & Willett, P. (2005). Use of graph theory to identify patterns of deprivation and high morbidity and mortality in public health data sets. Journal of the American Medical Informatics Association : JAMIA, 12(6), 630–641. https://doi.org/10.1197/jamia.M1714ArticlePubMedPubMed CentralGoogle Scholar
- Bendrey, R., & Martin, D. (2021). Zoonotic diseases: New directions in human-animal pathology. International journal of osteoarchaeology, 10.1002/oa.2975. Advance online publication. https://doi.org/10.1002/oa.2975
- Bhatia R. (2019). Implementation framework for One Health approach. The Indian journal of medical research, 149(3), 329–331. https://doi.org/10.4103/ijmr.IJMR_1517_18ArticlePubMedPubMed CentralGoogle Scholar
- Boots, M., & Sasaki, A. (1999). ‘Small worlds’ and the evolution of virulence: infection occurs locally and at a distance. Proceedings of the Royal Society of London. Series B: Biological Sciences, 266(1432), 1933–1938. ArticleCASPubMed CentralGoogle Scholar
- CDC: One Health Basics (accessed 27th May 2022 at https://www.cdc.gov/onehealth/basics/index.html and https://www.cdc.gov/onehealth/basics/zoonotic-diseases.html)
- Corcoran C. M. (2021). Commentary on “Lower speech connectedness linked to incidence of psychosis in people at clinical high risk”: The promise of graph theory and network neuroscience. Schizophrenia research, 228, 481–482. https://doi.org/10.1016/j.schres.2020.09.024ArticlePubMedGoogle Scholar
- Crépin, A. S., Karcher, M., & Gascard, J. C. (2017). Arctic Climate Change, Economy and Society (ACCESS): Integrated perspectives. Ambio, 46(Suppl 3), 341–354. https://doi.org/10.1007/s13280-017-0953-3ArticlePubMedPubMed CentralGoogle Scholar
- de Juan, S., Ospina-Alvarez, A., Villasante, S., & Ruiz-Frau, A. (2021). A Graph Theory approach to assess nature’s contribution to people at a global scale. Scientific Reports, 11(1), 1–13. Google Scholar
- Dos S Ribeiro, C., van de Burgwal, L., & Regeer, B. J. (2019). Overcoming challenges for designing and implementing the One Health approach: A systematic review of the literature. One health (Amsterdam, Netherlands), 7, 100085. https://doi.org/10.1016/j.onehlt.2019.100085ArticlePubMedGoogle Scholar
- Eames, K. T., & Keeling, M. J. (2003). Contact tracing and disease control. Proceedings. Biological sciences, 270(1533), 2565–2571. https://doi.org/10.1098/rspb.2003.2554ArticlePubMedPubMed CentralGoogle Scholar
- Edmunds, W. J., O'callaghan, C. J., & Nokes, D. J. (1997). Who mixes with whom? A method to determine the contact patterns of adults that may lead to the spread of airborne infections. Proceedings of the Royal Society of London. Series B: Biological Sciences, 264(1384), 949–957. ArticleCASPubMed CentralGoogle Scholar
- Éles, A., Heckl, I., & Cabezas, H. (2021). Modeling technique in the P-Graph framework for operating units with flexible input ratios. Central European Journal of Operations Research, 29(2), 463–489. ArticleGoogle Scholar
- Eteng, W. E., Mandra, A., Doty, J., Yinka-Ogunleye, A., Aruna, S., Reynolds, M. G., McCollum, A. M., Davidson, W., Wilkins, K., Saleh, M., Ipadeola, O., Manneh, L., Anebonam, U., Abdulkareem, Z., Okoli, N., Agenyi, J., Dan-Nwafor, C., Mahmodu, I., & Ihekweazu, C. (2018). Notes from the Field: Responding to an Outbreak of Monkeypox Using the One Health Approach - Nigeria, 2017–2018. MMWR. Morbidity and mortality weekly report, 67(37), 1040–1041. https://doi.org/10.15585/mmwr.mm6737a5ArticlePubMedPubMed CentralGoogle Scholar
- Eubank, S., Guclu, H., Kumar, V. S., Marathe, M. V., Srinivasan, A., Toroczkai, Z., & Wang, N. (2004). Modelling disease outbreaks in realistic urban social networks. Nature, 429(6988), 180–184. https://doi.org/10.1038/nature02541ArticleCASPubMedGoogle Scholar
- Friedler, F., Tarjan, K., Huang, Y. W., & Fan, L. T. (1992a). Combinatorial algorithms for process synthesis. Computers & chemical engineering, 16, S313–S320. 13 ArticleGoogle Scholar
- Friedler, F., Tarjan, K., Huang, Y. W., & Fan, L. T. (1992b). Graph-theoretic approach to process synthesis: axioms and theorems. Chemical Engineering Science, 47(8), 1973–1988. ArticleCASGoogle Scholar
- Friedler, F., Tarjan, K., Huang, Y. W., & Fan, L. T. (1993). Graph-theoretic approach to process synthesis: polynomial algorithm for maximal structure generation. Computers & Chemical Engineering, 17(9), 929–942. ArticleCASGoogle Scholar
- Friedler, F., Varga, J. B., & Fan, L. T. (1995). Decision-mapping: a tool for consistent and complete decisions in process synthesis. Chemical engineering science, 50(11), 1755–1768. ArticleCASGoogle Scholar
- Goicolea, T., Gastón, A., Cisneros-Araujo, P., García-Viñas, J. I., & Mateo-Sánchez, M. C. (2021). Deterministic, random, or in between? Inferring the randomness level of wildlife movements. Movement ecology, 9(1), 1–14. ArticleGoogle Scholar
- Gonzalez, A., Thompson, P., & Loreau, M. (2017). Spatial ecological networks: planning for sustainability in the long-term. Current opinion in environmental sustainability, 29, 187–197. ArticlePubMedPubMed CentralGoogle Scholar
- Hemida, M. G., & Ba Abduallah, M. M. (2020). The SARS-CoV-2 outbreak from a one health perspective. One health (Amsterdam, Netherlands), 10, 100127. https://doi.org/10.1016/j.onehlt.2020.100127ArticlePubMedGoogle Scholar
- Hueffer, K., Ehrlander, M., Etz, K., & Reynolds, A. (2019). One health in the circumpolar North. International journal of circumpolar health, 78(1), 1607502. https://doi.org/10.1080/22423982.2019.1607502ArticlePubMedPubMed CentralGoogle Scholar
- Hutchinson, Matthew & Bramon Mora, Bernat & Pilosof, Shai & Barner, Allison & Kéfi, Sonia & Thébault, Elisa & Jordano, Pedro & Stouffer, Daniel. (2018). Seeing the forest for the trees: Putting multilayer networks to work for community ecology. Functional Ecology. 33. https://doi.org/10.1111/1365-2435.13237
- Jeong, H., Tombor, B., Albert, R., Oltvai, Z. N., & Barabási, A. L. (2000). The large-scale organization of metabolic networks. Nature, 407(6804), 651–654. ArticleCASPubMedGoogle Scholar
- Keeling, M. J. (1999). The effects of local spatial structure on epidemiological invasions. Proceedings of the Royal Society of London. Series B: Biological Sciences, 266(1421), 859–867. CASGoogle Scholar
- Keeling, M. J., & Eames, K. T. (2005). Networks and epidemic models. Journal of the royal society interface, 2(4), 295–307. ArticlePubMedPubMed CentralGoogle Scholar
- Krämer, N., Schäfer, J., & Boulesteix, A. L. (2009). Regularized estimation of large-scale gene association networks using graphical Gaussian models. BMC bioinformatics, 10(1), 1–24. ArticleGoogle Scholar
- Kunhikannan, S., Thomas, C. J., Franks, A. E., Mahadevaiah, S., Kumar, S., & Petrovski, S. (2021). Environmental hotspots for antibiotic resistance genes. MicrobiologyOpen, 10(3), e1197. https://doi.org/10.1002/mbo3.1197ArticleCASPubMedPubMed CentralGoogle Scholar
- Lao, A., Cabezas, H., Orosz, Á., Friedler, F., & Tan, R. (2020). Socio-ecological network structures from process graphs. PloS one, 15(8), e0232384. https://doi.org/10.1371/journal.pone.0232384ArticleCASPubMedPubMed CentralGoogle Scholar
- Lakner, Rozália & Friedler, Ferenc & Bertók, Botond. (2017). Synthesis and Analysis of Process Networks by Joint Application of P-graphs and Petri Nets. 309–329. https://doi.org/10.1007/978-3-319-57861-3_18.
- Layeghifard, M., Hwang, D. M., & Guttman, D. S. (2017). Disentangling interactions in the microbiome: a network perspective. Trends in microbiology, 25(3), 217–228. ArticleCASPubMedGoogle Scholar
- Lebov, J., Grieger, K., Womack, D., Zaccaro, D., Whitehead, N., Kowalcyk, B., & MacDonald, P. (2017). A framework for One Health research. One health (Amsterdam, Netherlands), 3, 44–50. https://doi.org/10.1016/j.onehlt.2017.03.004
- Liu, Y., Gayle, A. A., Wilder-Smith, A., & Rocklöv, J. (2020). The reproductive number of COVID-19 is higher compared to SARS coronavirus. Journal of travel medicine. Google Scholar
- Ma, Z., Lee, S., & Jeong, K. C. (2019). Mitigating Antibiotic Resistance at the Livestock-Environment Interface:A Review. Journal of microbiology and biotechnology, 29(11), 1683–1692. https://doi.org/10.4014/jmb.1909.09030
- MacDonald, J. P., Ford, J. D., Willox, A. C., & Ross, N. A. (2013). A review of protective factors and causal mechanisms that enhance the mental health of Indigenous Circumpolar youth. International journal of circumpolar health, 72, 21775. https://doi.org/10.3402/ijch.v72i0.2177ArticlePubMedGoogle Scholar
- Maheswaran, R., Craigs, C., Read, S., Bath, P. A., & Willett, P. (2009). A graph-theory method for pattern identification in geographical epidemiology–a preliminary application to deprivation and mortality. International Journal of Health Geographics, 8(1), 1–8. ArticleGoogle Scholar
- Meyers, L. A., Pourbohloul, B., Newman, M. E., Skowronski, D. M., & Brunham, R. C. (2005). Network theory and SARS: predicting outbreak diversity. Journal of theoretical biology, 232(1), 71–81. ArticlePubMedGoogle Scholar
- Newman, M. E. (2002). Spread of epidemic disease on networks. Physical review E, 66(1), 016128. ArticleCASGoogle Scholar
- Nilsson, A., Bonander, C., Strömberg, U., & Björk, J. (2021). A directed acyclic graph for interactions. International journal of epidemiology, 50(2), 613–619. ArticlePubMedGoogle Scholar
- Park, J., Choi, J., & Choi, J. Y. (2021). Network analysis in systems epidemiology. Journal of Preventive Medicine and Public Health, 54(4), 259. ArticlePubMedPubMed CentralGoogle Scholar
- Pimentel, J., Orosz, Ákos, Tan, R. R., & Friedler, F. (2020). Automated Synthesis of Process-Networks by the Integration of P-graph with Process Simulation. Chemical Engineering Transactions, 81, 1171–1176. https://doi.org/10.3303/CET2081196ArticleGoogle Scholar
- Ruscio, B. A., Brubaker, M., Glasser, J., Hueston, W., & Hennessy, T. W. (2015). One Health – a strategy for resilience in a changing arctic. International journal of circumpolar health, 74, 27913. https://doi.org/10.3402/ijch.v74.27913ArticlePubMedGoogle Scholar
- Scharl, T., Voglhuber, I., & Leisch, F. (2009). Exploratory and inferential analysis of gene cluster neighborhood graphs. BMC bioinformatics, 10, 288. https://doi.org/10.1186/1471-2105-10-288ArticleCASPubMedPubMed CentralGoogle Scholar
- Schmiege, D., Perez Arredondo, A. M., Ntajal, J., Minetto Gellert Paris, J., Savi, M. K., Patel, K., Yasobant, S., & Falkenberg, T. (2020). One Health in the context of coronavirus outbreaks: A systematic literature review. One health (Amsterdam, Netherlands), 10, 100170. https://doi.org/10.1016/j.onehlt.2020.100170
- Seidl, R., Müller, J., Hothorn, T., Bässler, C., Heurich, M., & Kautz, M. (2016). Small beetle, large scale drivers: How regional and landscape factors affect outbreaks of the European spruce bark beetle. Journal of Applied Ecology, 53(2), 530–540. ArticleGoogle Scholar
- Sinclair J. R. (2019). Importance of a One Health approach in advancing global health security and the Sustainable Development Goals. Revue scientifique et technique (International Office of Epizootics), 38(1), 145–154. https://doi.org/10.20506/rst.38.1.2949ArticleCASPubMedGoogle Scholar
- Spencer, T. J., Thompson, B., Oliver, D., Diederen, K., Demjaha, A., Weinstein, S., Morgan, S. E., Day, F., Valmaggia, L., Rutigliano, G., De Micheli, A., Mota, N. B., FusarPoli, P., & McGuire, P. (2021). Lower speech connectedness linked to incidence of psychosis in people at clinical high risk. Schizophrenia research, 228, 493–501. https://doi.org/10.1016/j.schres.2020.09.002ArticlePubMedGoogle Scholar
- Szendroi, B., & Csányi, G. (2004). Polynomial epidemics and clustering in contact networks. Proceedings of the Royal Society of London. Series B: Biological Sciences, 271(suppl_5), S364–S366. Google Scholar
- Tompson, A. C., Manderson, L., & Chandler, C. (2021). Understanding antibiotic use: practices, structures and networks. JAC-antimicrobial resistance, 3(4), dlab150. https://doi.org/10.1093/jacamr/dlab150ArticleCASPubMedPubMed CentralGoogle Scholar
- Wang, W., Yang, X., Wu, C., & Yang, C. (2020). CGINet: graph convolutional network-based model for identifying chemical-gene interaction in an integrated multi-relational graph. BMC bioinformatics, 21(1), 1–17. ArticleGoogle Scholar
- World Health Organization (WHO): COVID-19 (accessed 27th May 2022 at https://www.who.int/emergencies/diseases/novel-coronavirus-2019/origins-of-the-virus) 16
- Zinsstag, J., Crump, L., Schelling, E., Hattendorf, J., Maidane, Y. O., Ali, K. O., Muhummed, A., Umer, A. A., Aliyi, F., Nooh, F., Abdikadir, M. I., Ali, S. M., Hartinger, S., Mäusezahl, D., de White, M., Cordon-Rosales, C., Castillo, D. A., McCracken, J., Abakar, F., Cercamondi, C., … Cissé, G. (2018). Climate change and One Health. FEMS microbiology letters, 365(11), fny085. https://doi.org/10.1093/femsle/fny085
- Yang, Y., Walker, T. M., Kouchaki, S., Wang, C., Peto, T., Crook, D. W., CRYPTIC Consortium, & Clifton, D. A. (2021). An end-to-end heterogeneous graph attention network for Mycobacterium tuberculosis drug-resistance prediction. Briefings in bioinformatics, 22(6), bbab299. https://doi.org/10.1093/bib/bbab299
- University of Alaska, Venn One Health diagram, accessed 31st May 2022 at https://www.uaf.edu/vmed/news-events/one-health/index.php
Acknowledgments
The author Ifigeneia Sideri acknowledges financial support for the dissemination of this work from the MSc “Bioinformatics and Neuroinformatics” program of Hellenic Open University.